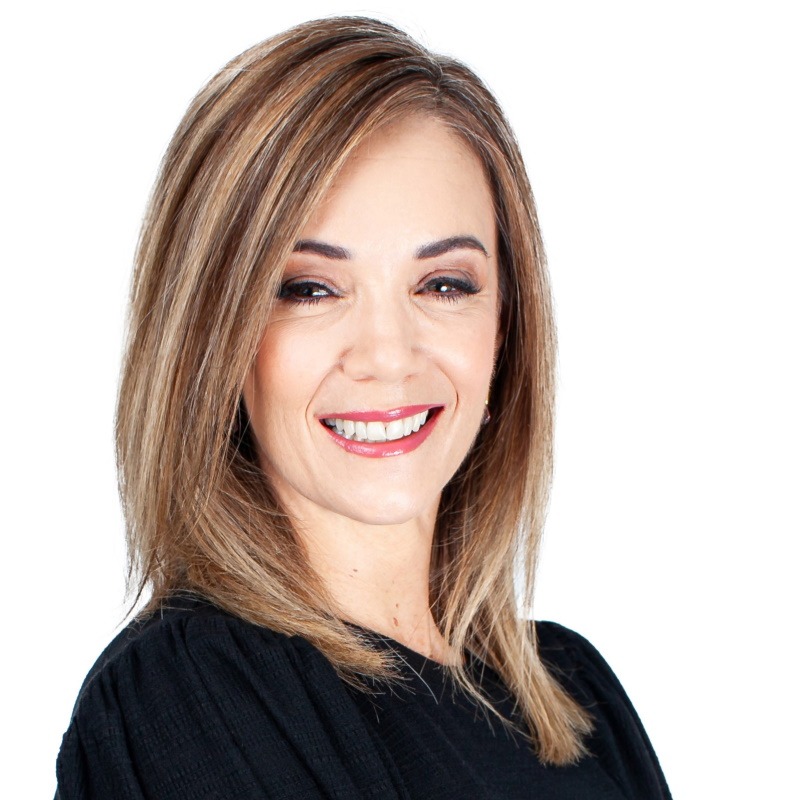
Generative Artificial Intelligence (AI) models have revolutionised various industries, from art and music creation to natural language processing. However, concerns are emerging regarding the size and integrity of training materials used for these models and the possibility of copyright infringement.
Ultraman court decision
It was only a matter of time before the world’s court systems would start to issue judgments on the infringement of copyright in materials used to train generative AI models, and China’s Internet Court in Guangzhou, is amongst the first.
This court heard a case centred around the title character of a Japanese animated TV show, a superhero named Ultraman. Tsuburaya Productions Co. Limited (Tsuburaya) is the owner of the copyright in works associated with the Ultraman series, which includes the Ultraman character. Tsuburaya granted Shanghai Character License Administrative Co. Limited (SCLA) an exclusive license in relation to this copyright in China, including the right to enforce the copyright against infringers and to create derivative works from the originals.
When it became apparent to SCLA that images generated by Tab - a generative AI service provided in China- bore a marked similarity to the original Ultraman character, SCLA sought recourse from the Internet Court.
According to Rules entitled “Interim Measures for the Management of Generative Artificial Intelligence Services”, effective in China since 1 January 2023, providers of AI services have an obligation to respect the intellectual property rights of others and “not to use advantages such as algorithms, data and platforms to engage in unfair competition”.
Applying these Rules to the Ultraman case, the Court held that the images generated by the Tab service are derivatives of the original artistic works (an act reserved exclusively for SCLA in China) and, therefore, that they constitute an infringement of the copyright subsisting in these original works.
Size and integrity of AI training data
This case has highlighted the importance of using sufficient, accurate, and unbiased content to train generative AI models. The adage "garbage in, garbage out" couldn't be more apt.
Good AI models are trained on massive amounts of data - and quality is equally important. Training an AI model is analogous to teaching a child to recognise objects. If the child is exposed to accurate and diverse examples, they develop a robust understanding. Similarly, AI models require a rich, varied, and representative dataset to comprehend patterns and generalise effectively.
Bias is a key concern when it comes to AI training data. If the dataset is skewed or incomplete, the AI model will inherit those biases in its outputs, potentially perpetuating and even amplifying them. This can have real-world consequences, from discriminatory outcomes to copyright infringement.
The European Union’s regulations for the development of ethical AI systems requires providers to ensure that training, validation and testing data sets are relevant, representative, free of errors and complete. The data sets must have the appropriate “statistical properties, met at the level of individual data sets or a combination thereof”. Put simply, to generate reliable, useful and lawful outputs, an AI model must be trained on a statistically significant set of accurate and representative content.
A phenomenon known as “overfitting” can occur when a model is incapable of generalising - instead, its outputs align too closely to the training dataset. Overfitting is likely if:
- The size of the training dataset is too small,
- It contains large amounts of irrelevant information (“noise”),
- The model trains for too long on a single sample set of data, or
- The model is complex, so it inadvertently learns the noise within the training data.
Essentially, when an AI model becomes too specialised in the training data, it fails dismally at generalising and applying knowledge to adapt to new, unseen examples.
Overfitting can also lead to “hallucinations”, which is where the model perceives patterns or objects that do not exist or are imperceptible to human observers, producing outputs that are nonsensical or just plain wrong. If an AI model is trained on a dataset containing biased or unrepresentative data, it may hallucinate patterns or features that reflect these biases.
To understand how this happens, consider the manner in which Large Language Models (LLMs) are trained: training text is broken down into smaller units, called tokens (which can be a word or even a letter, for example).In training, a sequence of tokens are provided to an LLM and it is required to predict what the next token will be. The output is then compared to the actual text, with the model adjusting its parameters each time to improve its prediction. An LLM never actually learns the meaning of the words themselves, which means that it can generate outputs that, from a language point of view, are wonderfully fluent, plausible and pleasing to the user but are nevertheless factually inaccurate.
Infringement of copyright in training material
To avoid the problems associated with improper training of AI models, hundreds of gigabytes of relevant and error-free training content must be assembled from trustworthy sources. So, it is not surprising that we have seen a recent flurry of lawsuits claiming the unauthorised use or reproduction of copyright works by generative AI service providers. Probably most famously, the New York Times instituted proceedings against Microsoft and Open AI for reproducing and using its articles without permission in the generative AI services offered by Microsoft's Co-Pilot (formerly Bing Chat) and OpenAI's Chat GPT. NYT claims that the defendants are using the fruits of its “massive investment in journalism”, to enable its models. To make matters worse, some of the training content was only made accessible by NYT to its subscribers in exchange for a fee. Despite being protected by a paywall, the content is being used by AI models to produce outputs that are accessible to all those with internet connectivity.
Fair dealing or unfair usage?
Generally, the trend is for AI service providers to claim fair use (also known in South Africa as fair dealing) as a defence to an allegation of copyright infringement. Fair use is a legal rule in copyright law that permits the use of original copyright works without the authorisation of the copyright owner, in certain ways and for certain purposes, with the aim of balancing the rights of the copyright owner with the rights of others to use and enjoy the original works.
With AI models, needing big datasets for training, fair use has become a big deal. As Court decisions to guide us on the approach likely to be taken when it comes to fair use in the context of AI, are still scarce. We expect that a key factor will be whether or not the use of original copyright works is "transformative." In other words, does using the original works contribute to the creation of something new or different? In AI training, this happens when copyright works are used to find new insights, patterns, or knowledge, instead of merely copying these.
In an ideal world, generative AI outputs would not bear an objective similarity to any one item of training material – the model would use these to itself generate original works influenced by all of the available training material. The reality is that failing to train an AI model responsibly may produce outputs that too closely resemble the training material, resulting in copyright infringement.
Conclusion
As the use of generative AI models has become more widespread, the need for industry standards, guidelines and best practices for training content, has become increasingly evident. Collaborative efforts within the AI community and from legal experts and policymakers, are essential to address the challenges associated with training effectiveness and to facilitate responsible and ethical use of generative AI models.
Share